Introduction
Human settlements represent the footprints of human activity on the planet. The ability to detect and attribute them automatically helps us unlock insights about how civilization is evolving, which communities are thriving and where investment can fuel sustainable development.
Atlas AI uses proprietary AI models to fuse a range of input data sources at varying spatial resolutions to detect, extract and analyze human settlements globally, at scale and with very high precision.
Knowledge of human settlements combined with other socioeconomic indicators helps us analyze the level of prosperity, and the needs, risks, and growth trends of local communities in any part of the world.
Human settlements are spatial constructs that do not conform to a single definition. They are aggregations of all registered built-up structures protruding from the ground. At the finest aggregation level human settlements estimate individual built-up structures, a geospatial layer that can be thought of as a global built-up base-map that we refer to as the Atlas of Human Settlements (AHS).
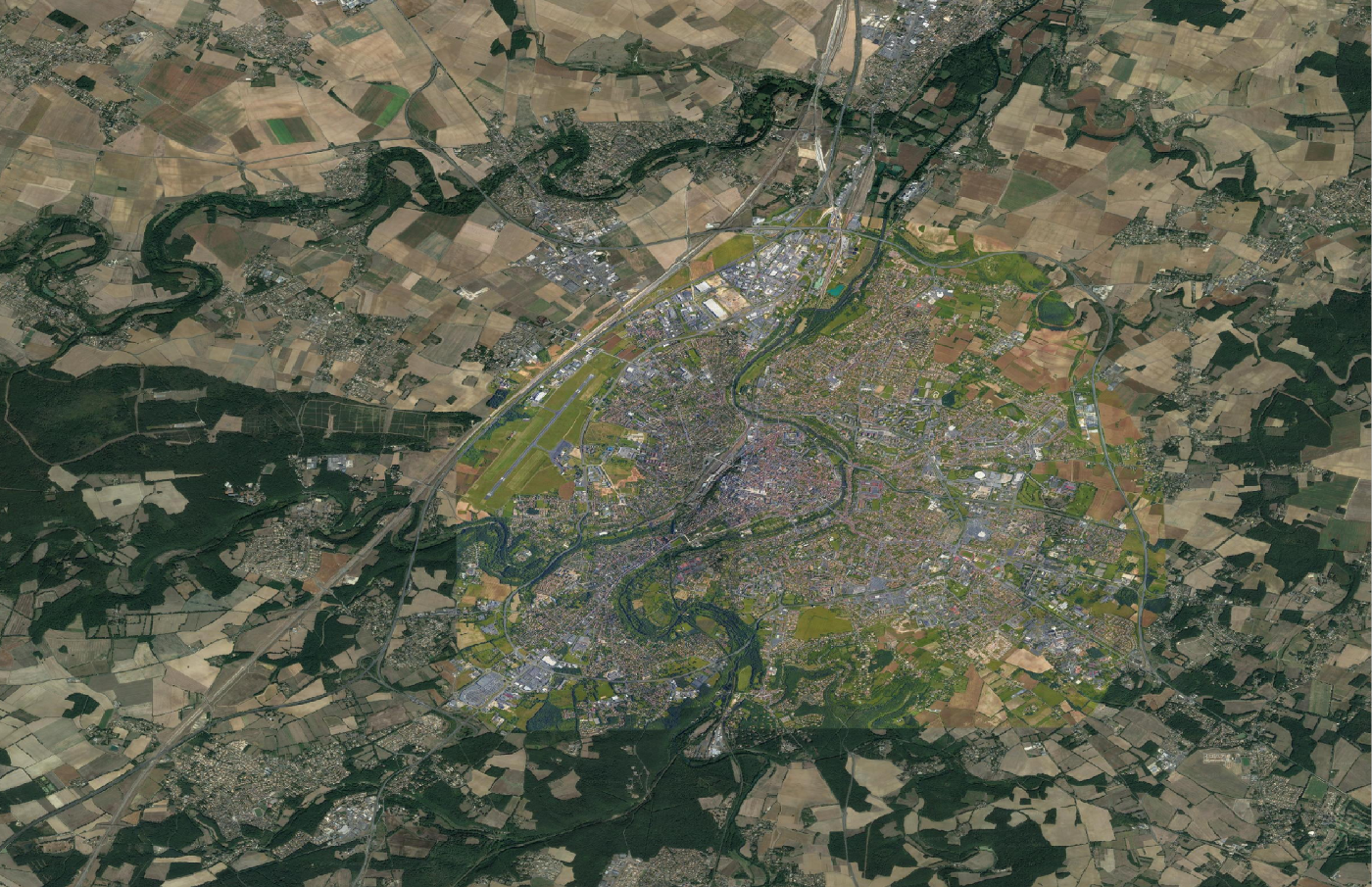

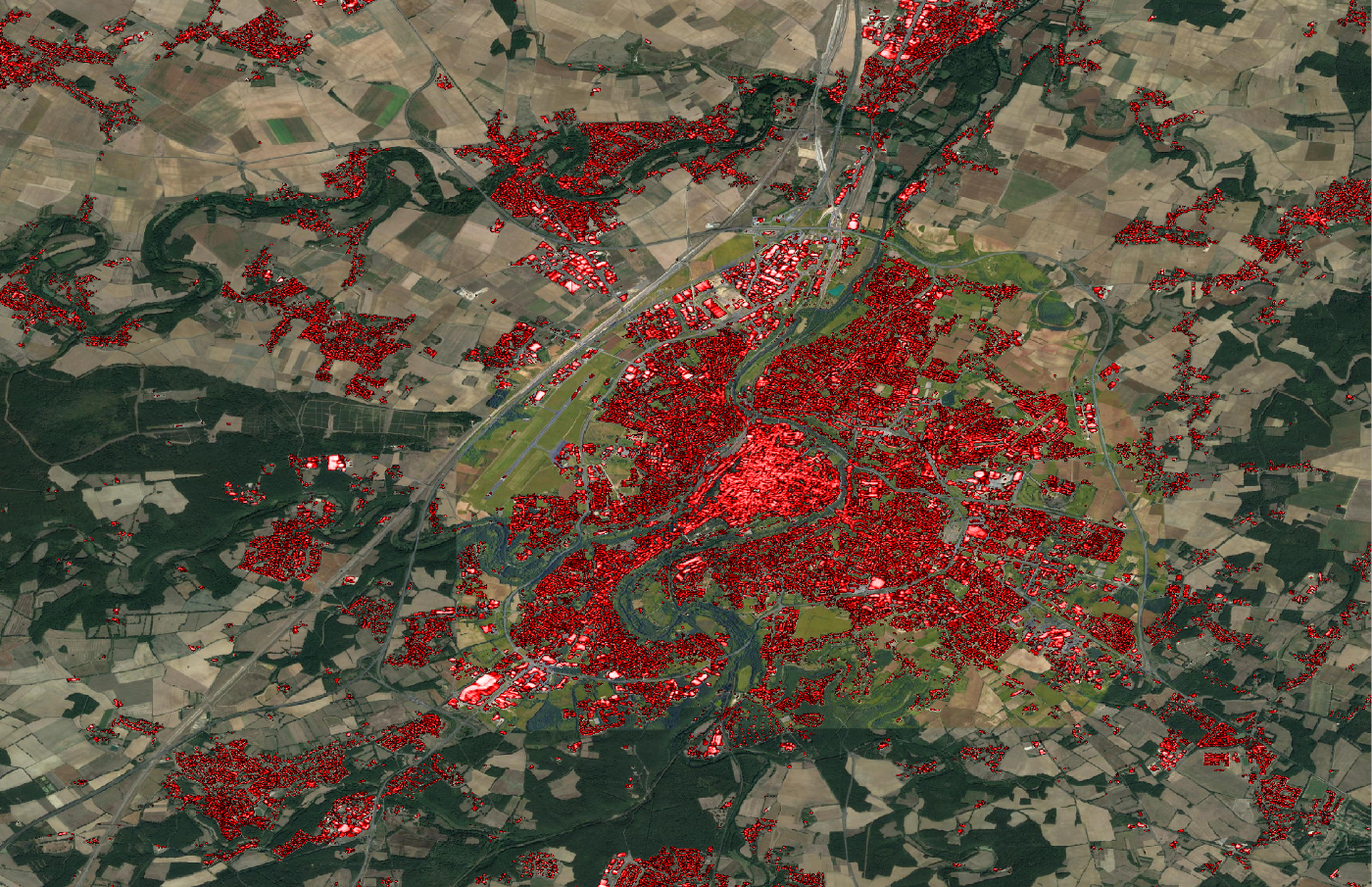
Increasing the spatial generalization scale leads to wider aggregations and thus coarser definitions of settlements that can be tuned to application-specific requirements.
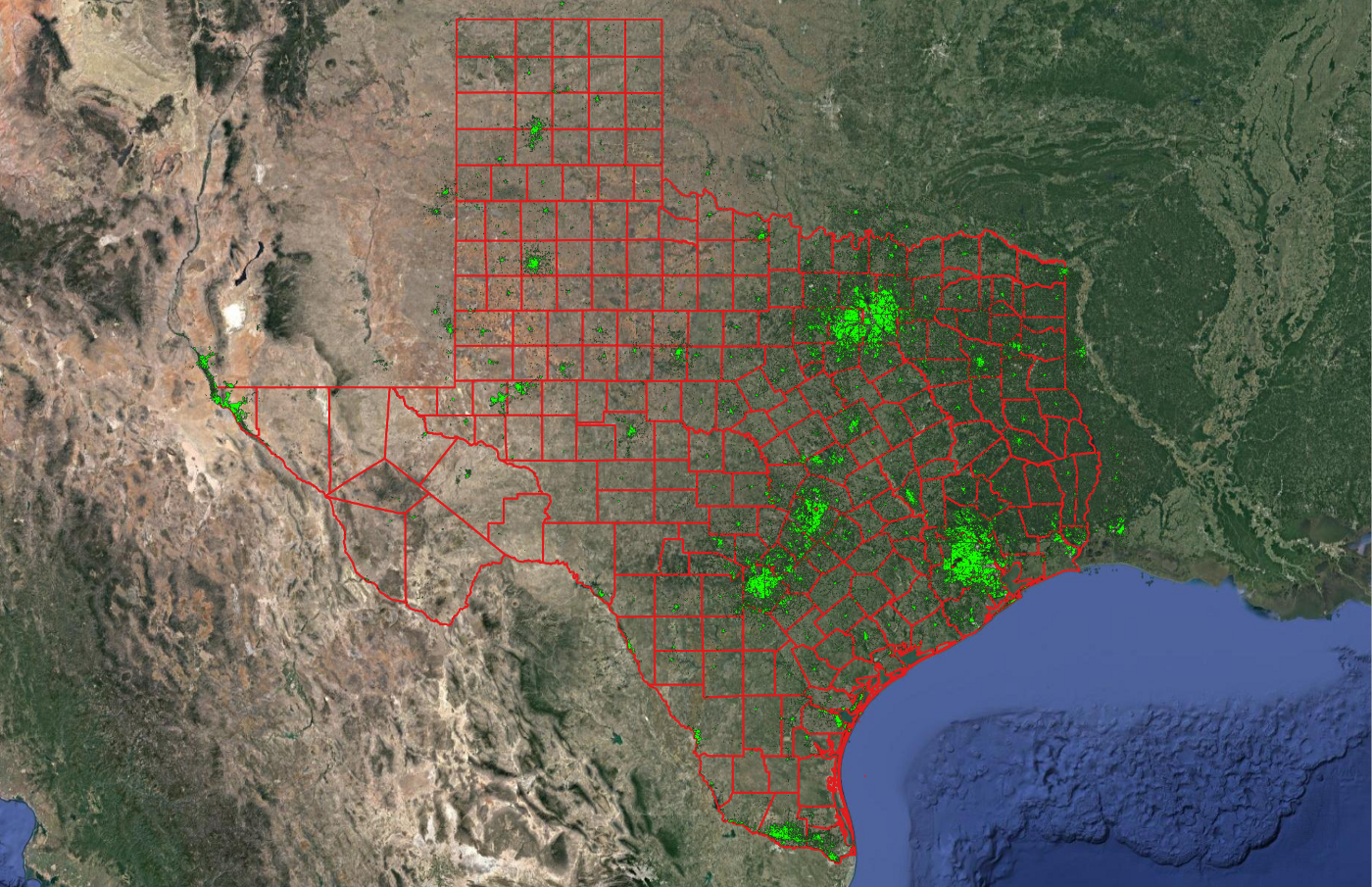


Human settlements can be attributed from a pool of inherent features. Typical settlement attributes include:
- structural attributes (size, perimeter, linearity, compactness, boundary smoothness, etc.),
- building count (resolution permitting),
- built-up surface and density,
- building size distribution,
- built-up labels, e.g. residential vs non residential, commercial vs industrial, etc.
Settlement attributes are used to instantiate the settlement ontology, which finds use in machine learning applications and analytics workflows that aid us in our primary mission, to model socioeconomic conditions at a global scale.
Technology — Delivery
The production of AHS involves a delicate orchestration of state of the art technologies from the fields of artificial intelligence, data science/engineering, computer vision and remote sensing. Assembling sophisticated workflows that are hosted and powered by the industry’s leading cloud service provider, Google Cloud Platform (GCP), and using the robustness and scalability of the Google Earth Engine (GEE), we produce, update and maintain the collection of AHS data layers in annual intervals with the possibility of custom releases upon demand.
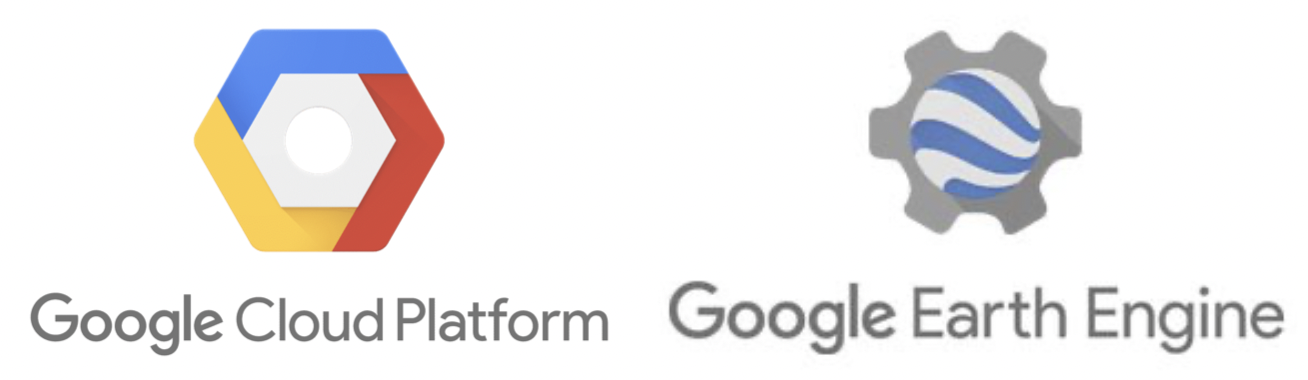
At the core of our technology there exists a single, custom-designed, deep encoder-decoder network that is trained and regularly updated from vast data collections that image habited regions of the world. The imagery used for the production of the AHS is the global Sentinel-2 annual composite mosaic. The AHS model is trained from tens of millions of built-up instances and bears knowledge of the types, patterns, shapes, sizes and texture of built-up, that is transferable to any geography for which built-up needs to be inferred.
AHS is the single most complete global built-up base-map of the world that meets the quality criteria set forth by the Joint Research Center of the European Commission in the call for tenders titled ‘Global Human Settlement Layer in the Copernicus Emergency Management System’.
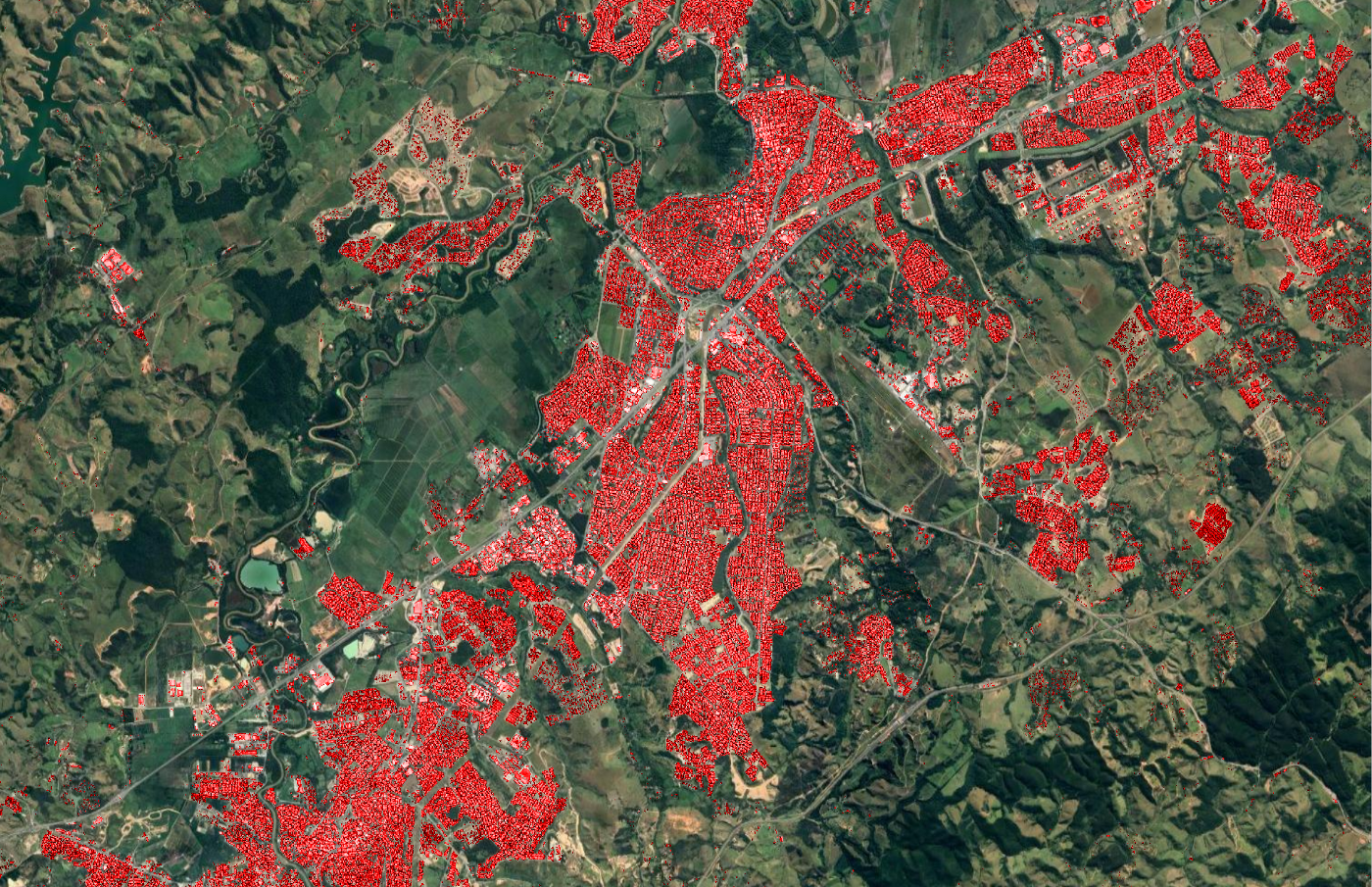
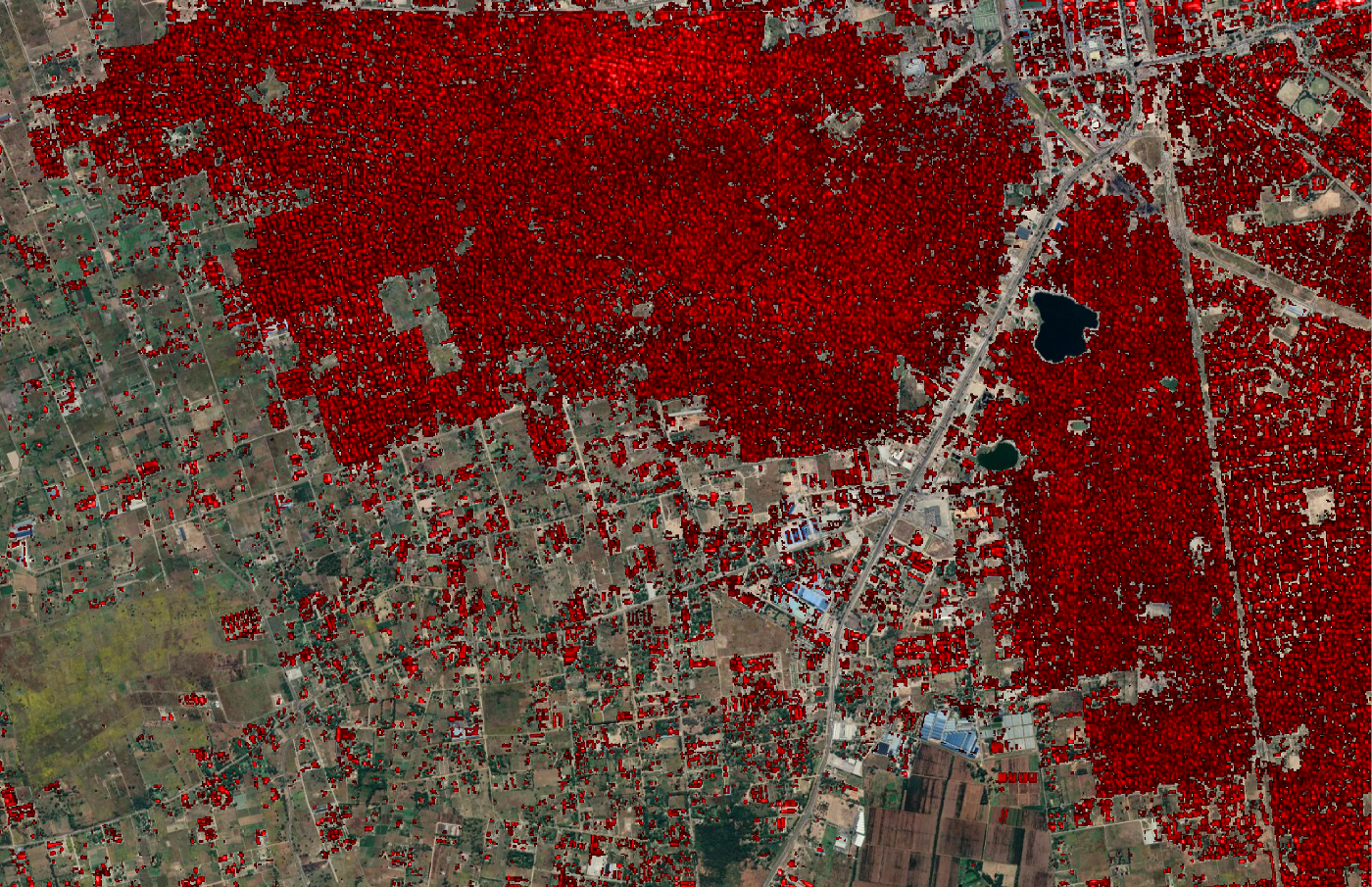
State of the Product
AHS layers are produced incrementally. During this process, strict regional content controls guarantee a globally consistent deliverable that meets the industry’s quality standards.
AHS-2021 is currently in beta release with selected geographies made publicly available for evaluation purposes. Public data include the pre-release of:
- 2021 Built-up Surface (BuS), and the
- 2021 Built-up Index (BuI).
The BuS layer is a built-up confidence map with extreme values in the range of 0.0, and 1.0 (100%). The layer is subject to a threshold operation that delivers a binary raster, through the application of automatically derived, region-specific threshold values.
The BuI layer is the built-up index with extreme values in the range of 0.0, and 1.0 (100%). Pixel intensity specifies the ratio of built-up surface (m2) to the pixel surface (m2). The layer is subject to a masking operator using the binary BuS layer as an acceptance mask.
The layers to be released include the:
- 2021 binary BuS, and the
- 2021 masked BuI.
Beta Release Layer Samples
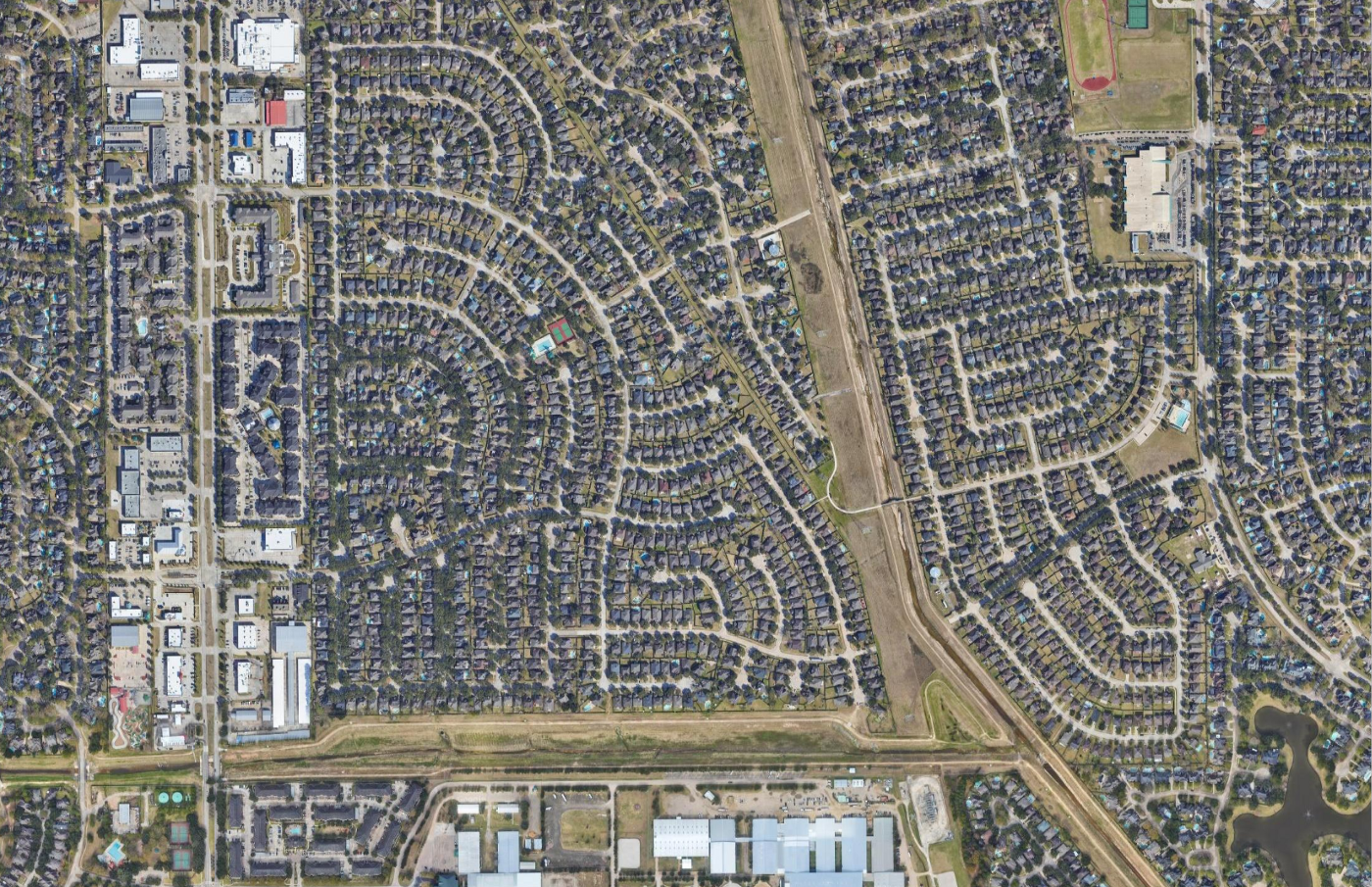

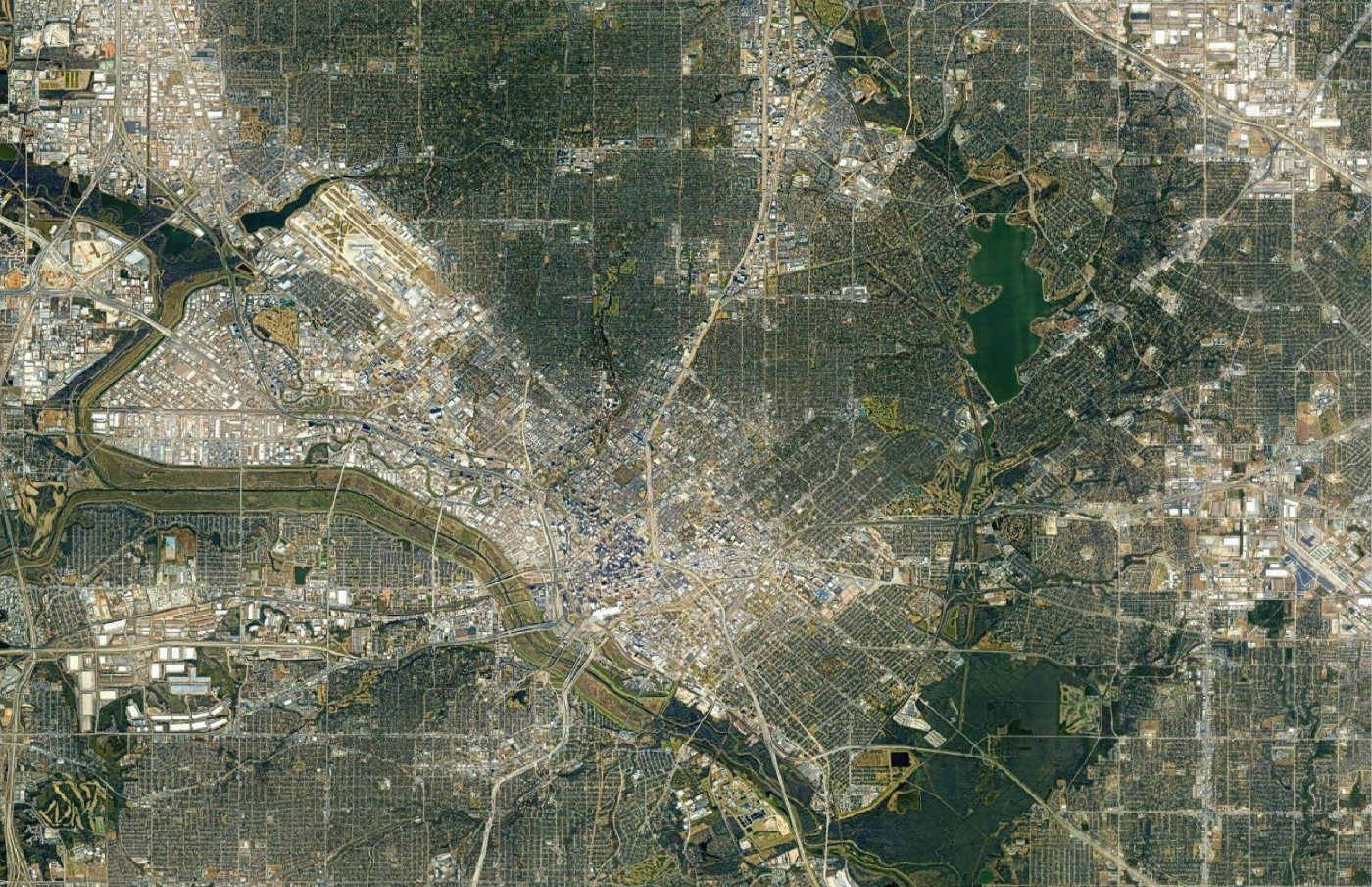
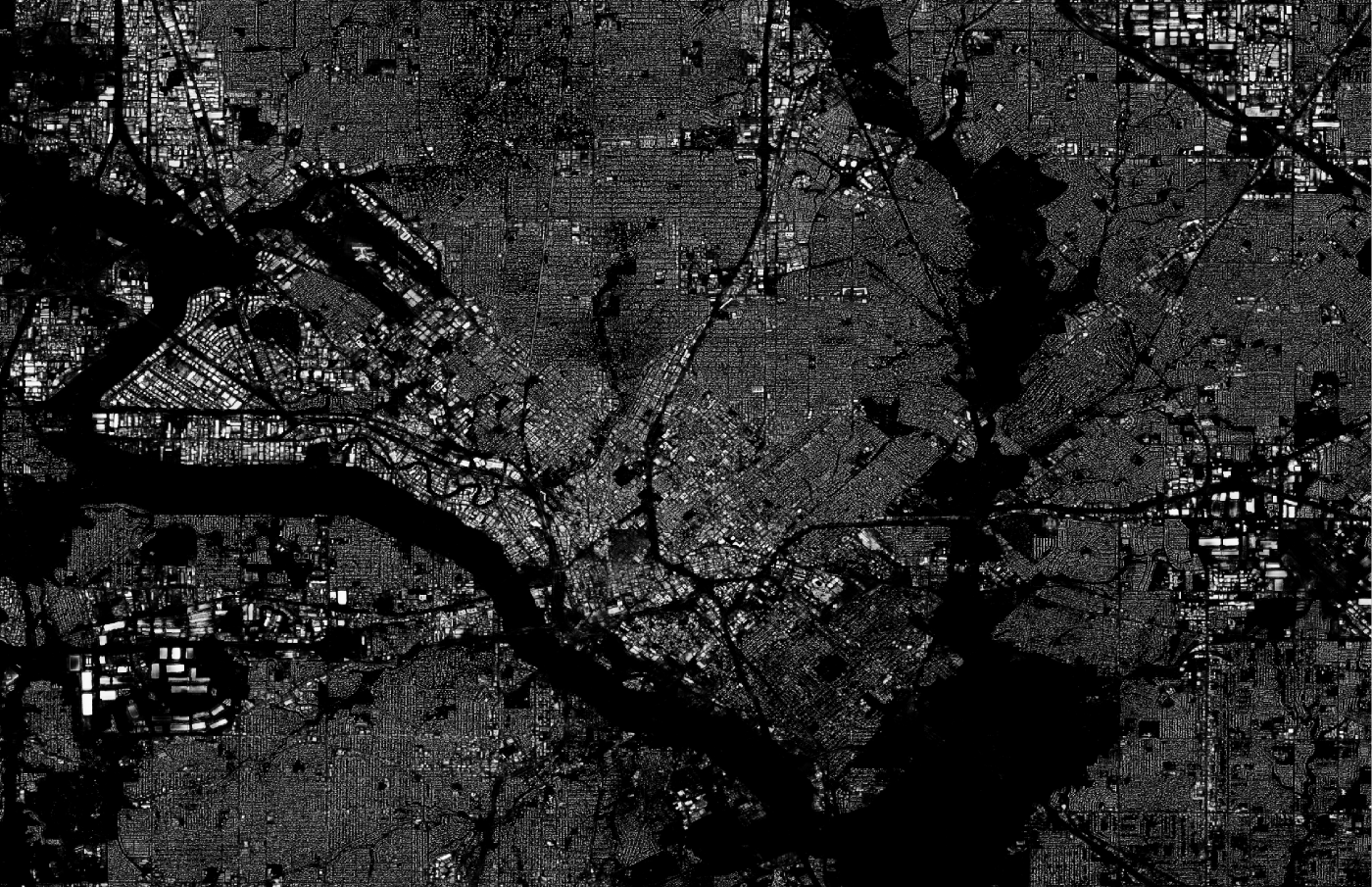
Sample layers are made publicly available for evaluation purposes only. Follow the hyperlinks to access the 2021 BuS and BuI pre-release layers inferred over Texas, USA. Evaluation AHS data layers and supporting materials are open to the community under CC BY-NC-SA 4.0 license terms.
Both layers have been generated by a USA-focused submodel. The table below lists indicative model metrics on the validation and test sets used.

Contact us
We are eager to collaborate with the research community and with individuals, non-profit organizations and corporations seeking to use the Atlas of Human Settlements in your work. To submit research proposals for free beta access to the AHS, or to explore commercial use of the data contact us at info@atlasai.us.